Why Your AI Is Failing Customers — And How Model Context Protocol (MCP) Fixes It
Imagine a brilliant library assistant who can answer questions quickly, but has no long-term memory and can only view a few books at once. With each new question, they must guess which references to use, often missing critical context from your previous requests.
Now imagine this assistant has a specialized notebook that automatically records key information from every conversation. Instead of starting from scratch each time, they can reference this notebook to maintain continuity, while you control which information is most important to remember.
This is what Model Context Protocol (MCP) does for AI systems, providing the foundational structure that makes AI collaboration truly effective.
What Is Model Context Protocol?
Model Context Protocol (MCP) is a framework that enhances how AI systems manage and retain context throughout user interactions by organizing information into three distinct layers:
- System Context: Stable instructions that define the AI's core behavior and capabilities
- User Context: Continuously evolving insights into user preferences, goals, and history
- Conversation Context: The real-time, dynamic exchange between the user and the AI
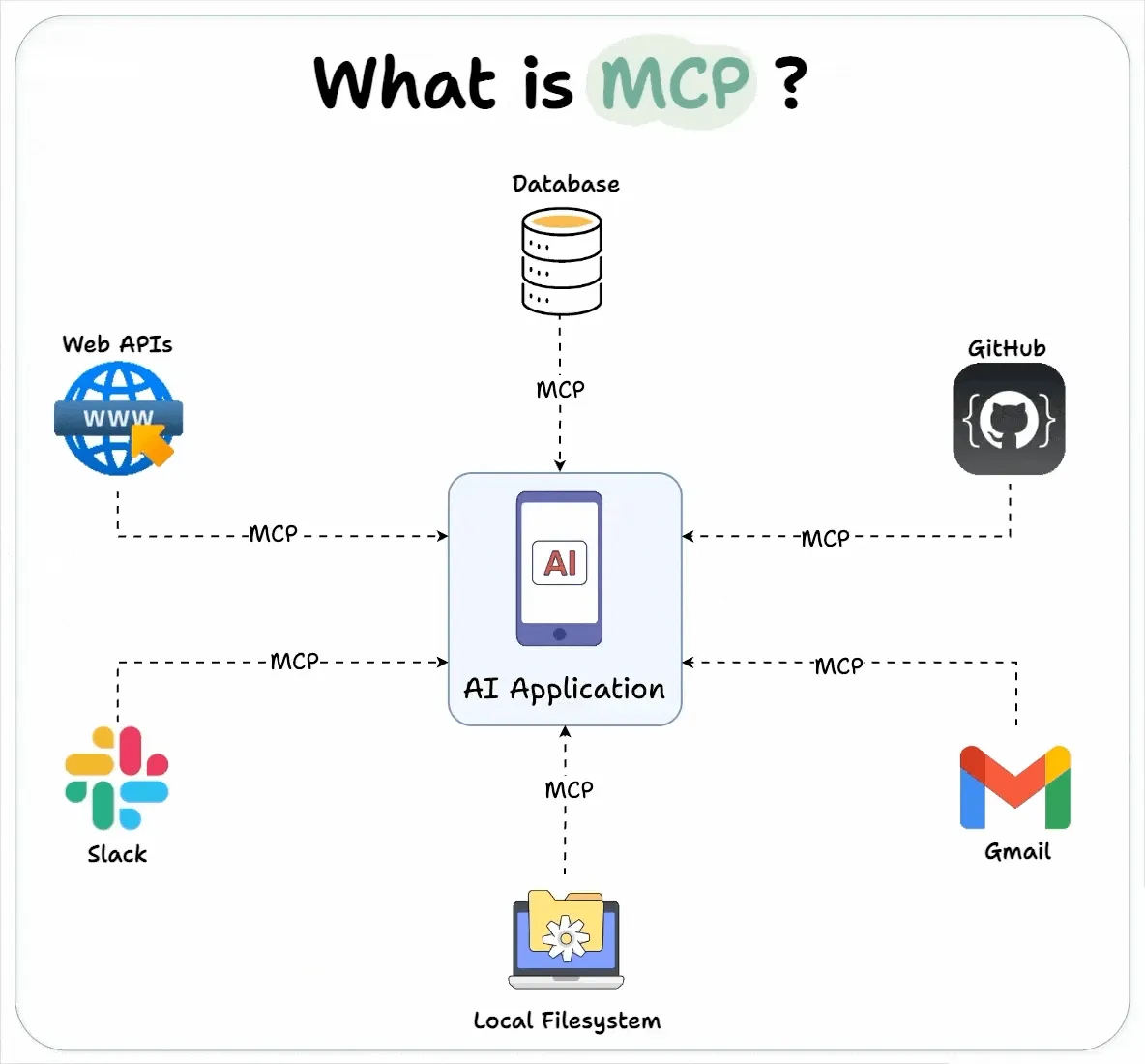
MCP connects AI applications to various data sources and services including databases, APIs, and collaboration tools
This structured approach allows AI systems to prioritize, retain, and reuse relevant information, leading to more coherent, intelligent, and personalized responses.MCP streamlines data integration by standardizing how AI applications connect to external tools and repositories
Business Challenges MCP Addresses
Customer Frustration & Repetitive Explanations
Customers often grow frustrated when AI systems forget past interactions, forcing them to re-explain issues — hurting customer satisfaction and brand perception.
Escalating Operational Costs
Without clear context boundaries, AI systems waste resources processing redundant or irrelevant data, driving up cloud and engineering costs.
Low Conversion and Resolution Rates
AI assistants frequently fail to recommend the right solution or product when context is missing, leading to poor conversion metrics and ineffective support resolutions.
How MCP Solves These Challenges
Strategic Prioritization
MCP ensures essential context persists throughout interactions, while low-priority data is summarized or removed to optimize performance.
Persistence Architecture
With MCP, context isn't lost between sessions — enabling consistent, personalized experiences over time.
Efficient Resource Allocation
By separating static system instructions from dynamic user and conversation data, MCP reduces processing overhead and improves response relevance.
Limitations of MCP
While MCP offers clear strategic value, its implementation does present some challenges:
Implementation Complexity
Structuring data across context layers requires planning, governance, and technical expertise.
Initial Setup Investment
Getting MCP right demands collaboration across business, data, and engineering teams — and upfront configuration time.
Data Classification Challenges
Organizations lacking well-structured data may struggle to define what goes into each context layer effectively.
Final Thoughts: How SEEN Can Help
Model Context Protocol (MCP) is a game-changer for businesses looking to scale AI systems without sacrificing intelligence or user experience. Its structured approach to context unlocks better customer support, higher conversions, and smarter automation.
At SEEN, we help organizations implement MCP with excellence and efficiency — offering tailored strategies, integration support, and cost-effective execution. Whether you're exploring MCP for the first time or refining your AI stack, SEEN is your trusted partner in building smarter, more sustainable AI solutions.